The maintenance of complex systems and machinery plays a critical role in ensuring their optimal performance and longevity. However, traditional approaches to maintenance, such as reactive or preventive strategies, are often limited in their ability to address failures and breakdowns effectively. This has led to the emergence of predictive maintenance, a proactive and data-driven approach that leverages advanced analytics and prognostic methods to predict and prevent potential failures before they occur. In this blog, we will delve into the fascinating world of prognostic methods in predictive maintenance applications, exploring four key methodologies: knowledge-based prognostic, data-driven prognostic, hybrid models, and physics-based prognostics. By understanding the principles and benefits of these methods, we can unlock new possibilities for enhancing operational efficiency, minimizing downtime, and maximizing the lifespan of critical assets.
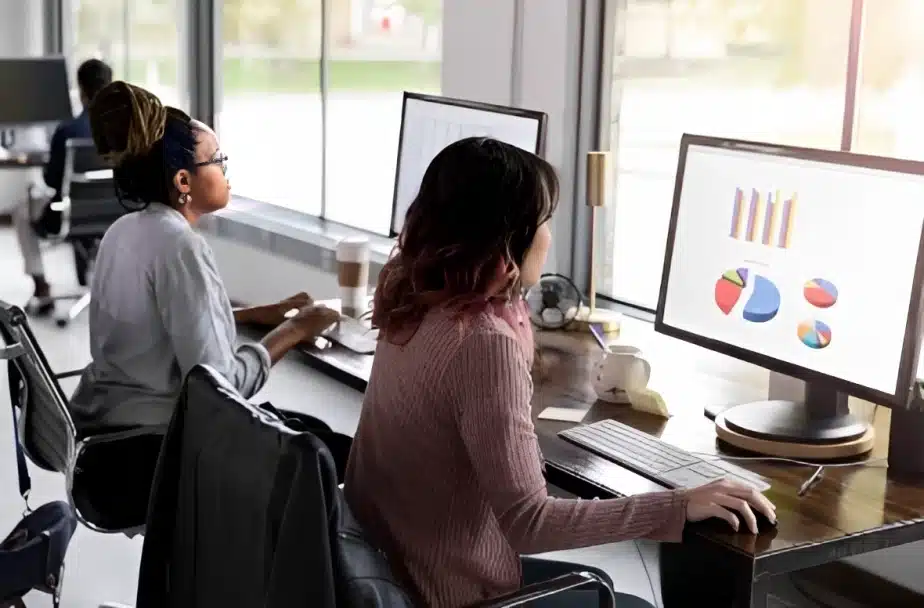
Knowledge-Based Prognostics
Knowledge-based prognostics relies on expert knowledge and domain expertise to predict the future behavior and health of systems or machinery. By leveraging existing knowledge, rules, and understanding of the system’s physics and historical performance data, prognostic models estimate the anomaly condition of machineries and forecast potential root-cause of failures. In the case of an aircraft engine, this approach utilizes information about the engine’s design, material properties, operating parameters, and historical performance to assess its health. By considering factors like temperature, pressure, vibration levels, and failure modes, the expert or analyst can predict the degradation and remaining life of critical components. Real-time data analysis and comparison with established rules enable maintenance teams to proactively schedule repairs or replacements, reducing the risk of unexpected failures.
Human operators and maintenance personnel contribute their expertise to knowledge-based prognostics, further refining predictions and enhancing accuracy. Their insights are integrated into the prognostic models, making them more robust. This approach proves valuable in industries such as aerospace, manufacturing, energy, and transportation, where complex systems require effective health management. By combining domain knowledge with advanced analytics, knowledge-based prognostics offers a powerful method for predicting and managing the health of various systems, minimizing downtime, and optimizing maintenance activities.
Data-Driven Prognostic Methods
Data-driven prognostics is a methodology that relies on analyzing historical data to predict the future behavior and health of a system or machinery. Instead of relying solely on expert knowledge, data-driven prognostics employs machine learning and statistical techniques to discover patterns, trends, and relationships within the data. By training predictive models on past data, these methods can accurately predict the remaining useful life (RUL) and potential failures of the system.
In the case of a fleet of wind turbines, data-driven prognostic methods involves collecting and analyzing sensor data such as temperature, vibration, wind speed, and power generation. Machine learning algorithms are then applied to uncover patterns and correlations that indicate the health and degradation of critical components. For example, the model may identify specific temperature fluctuations or vibration levels that precede failures in turbine bearings. By continuously monitoring real-time sensor data and comparing it to learned historical patterns, the model can make predictions about the RUL of the bearings, enabling proactive maintenance scheduling to minimize downtime.
Data-driven prognostics can incorporate diverse data sources beyond sensors, including maintenance logs, environmental conditions, and operational parameters. By integrating multiple data streams and applying advanced analytics techniques, the model captures complex relationships and provides more accurate predictions about system health and future failures.
An important advantage of data-driven prognostics is its adaptability and continuous improvement. As new data becomes available, the model can be retrained and refined, incorporating the latest information to enhance its predictive capabilities. This iterative learning process ensures ongoing improvement in prognostic accuracy and empowers maintenance teams to make data-driven decisions that optimize asset performance and maintenance activities.
Challenges with “Big Data”
In recent times, there has been excitement surrounding the use of statistical processing of big data in machine health monitoring. However, there are challenges associated with accessing complete life data of critical and expensive machines due to practical constraints and economic viability. For instance, machines cannot be continuously stopped and inspected throughout their fault development, and it is not feasible to allow a sufficient number of machines to fail to gather adequate data for big data techniques. Aircraft engines serve as a typical example, where the number of fatal accidents caused by mechanical failure is relatively small compared to the vast amount of flights. Moreover, it is impractical to collect statistical amounts of data on each potential failure type when considering the numerous components within an engine. Despite these limitations, big data can be valuable in analyzing machines in healthy or near healthy conditions to distinguish between actual condition and operating conditions that can affect vibration responses.
A potential solution to address these challenges lies in using simulation models to generate data from simulated faults in various machines and components. This approach allows for flexibility in varying the type, location, and severity of faults as desired, rather than relying on real faults to occur. Various faults in rotors, such as unbalance, misalignment, cracks, and rub, can be successfully modeled. Simulation models can be updated to match measurements using a limited amount of data and neural networks can be trained based on simulated responses. Examples have demonstrated the success of this approach in recognizing actual faults and providing estimations of fault severity. While this approach can be considered physics-based when the root cause of the fault is directly modeled, there are situations where the line between physics-based and data-driven approaches becomes blurred. For instance, when a physics-based model generates data corresponding to fault symptoms, such as crack development, and the information needs to be extracted similar to real measured data. Simulation of responses to localized and extended faults in bearings has also yielded positive results. However, introducing a realistic level of variability into simulated responses is necessary, as simulation models tend to be deterministic. This can be achieved by incorporating random variations based on measurements taken from the same engine at different times.
Hybrid Models
Hybrid models in prognostic methods combine the strengths of knowledge-based and data-driven approaches to achieve more accurate and robust predictions. These models integrate expert knowledge, domain expertise, physics-based models, and advanced analytics to improve prognostic outcomes. The knowledge-based component incorporates domain-specific rules and features, capturing the underlying principles of the system. The data-driven component utilizes historical data and machine learning algorithms to identify patterns and correlations that may not be explicitly captured by the knowledge-based component.
For instance, in manufacturing process failure prediction, a hybrid model may combine physics-based models describing degradation mechanisms with data-driven techniques learning from sensor data. The physics-based component provides insights into fundamental degradation processes, while the data-driven component captures complex interactions within the system. By combining these approaches, the hybrid model leverages the strengths of both methods and adapts to changing conditions, providing more accurate predictions for complex systems with limited data or uncertain physics.
The integration of knowledge-based and data-driven approaches in a hybrid model enhances accuracy, robustness, and adaptability in prognostics. It enables better decision-making, optimized maintenance planning, and improved asset performance across industries like manufacturing, energy, transportation, and healthcare. Hybrid models offer a comprehensive framework for prognostics by leveraging both expert knowledge and data-driven insights, allowing for more effective management of system health and proactive maintenance strategies.
Physics-Based Models
Physics-based prognostics, also known as model-based prognostics, involves using an in-depth understanding of the fundamental physics and principles of a system to predict its future behavior and health. These models are built on physical equations and mathematical models that govern the system’s operation, considering factors such as material properties, structural dynamics, forces, vibrations, and other physical phenomena. By incorporating real-time operating conditions, physics-based prognostics can estimate the remaining useful life (RUL) and identify potential failure modes.
In the automotive industry, physics-based prognostic methods can be applied to predict brake pad wear. By considering parameters like vehicle speed, brake pad material properties, temperature, and friction coefficients, the model simulates the frictional behavior and estimates the rate of wear. Monitoring the operating conditions and comparing them with the model’s predictions allows maintenance teams to proactively schedule brake pad replacements before critical wear levels are reached.
In power grids, physics-based prognostics utilize models based on electrical circuit theory, load dynamics, and component aging to predict the health and failure modes of critical components like transformers or circuit breakers. Factors such as voltage fluctuations, load patterns, and temperature are considered to estimate component degradation and predict the time until failure. This enables utility companies to plan maintenance activities, optimize infrastructure replacement, and prevent unexpected outages.
Physics-based prognostics offer insights into degradation mechanisms, adaptability to different conditions, and the ability to predict failures even without historical data. However, they require detailed system knowledge, accurate modeling, and precise parameter estimation. By combining physics-based models with real-time data monitoring and calibration, these prognostic methods improve maintenance decision-making, reduce downtime, and optimize asset performance in various industries.
Conclusion
In conclusion, prognostic methods in predictive maintenance offer various methodologies for failure prediction in complex systems. Knowledge-based prognostics utilize expert knowledge, data-driven prognostics employ historical data and machine learning, hybrid models combine knowledge-based and data-driven approaches, and physics-based prognostics rely on understanding physical principles.
Each method has its strengths and applications. Knowledge-based prognostics provide insights when data is limited or domain knowledge is crucial. Data-driven prognostics excel at capturing complex patterns, adapting to change, and continuous learning. Hybrid models balance domain expertise and data-driven insights for improved accuracy and robustness. Physics-based prognostics offer deep understanding and prediction even without historical data.
By leveraging these methods, industries can proactively identify failures, optimize maintenance, and extend asset lifespan. Integration of these methodologies has the potential to revolutionize predictive maintenance, enhancing efficiency, reducing downtime, and cutting costs. As technology advances, prognostics will continue evolving, unlocking new possibilities for asset management and improving system performance.
Recommended Blog Posts
September 4, 2023
Powerful Signal Analysis Tools for Vibration Analysis
Predictive maintenance, crucial for machinery reliability, heavily relies on vibration analysis. Techniques like FFT…
September 4, 2023
Rotating Machinery Vibration Analysis
Vibration analysis is a critical tool in various industries like manufacturing, power generation, and transportation.…
December 28, 2022
Fault Diagnostic Technique Using Machine Mode Similarity Analysis
AI can diagnose machine faults with vibration data but machine mode similarity analysis is an alternative, it uses…
September 15, 2022
Understanding Rotating Machinery Data
Machine data is generated by physical attributes and actions of machines, collected by sensors and analyzed for…
August 6, 2021
Envelope Analysis
Bearings are critical elements in rotating machines, they support radial and axial loads, and reduce friction. Real…
May 6, 2021
What is Cepstral Analysis?
Cepstral Analysis, a tool used to detect periodicity in frequency spectrum, can be useful in gearbox fault detection in…
October 9, 2020
How is Fault Detection Performed?
Vibration measurements and analysis, using multiple parameters, can identify developing problems in machinery before…
September 21, 2020
Parameter Selections in Vibration Measurement
Vibration measurements are used to determine the response of machines to forces and identify potential issues. It is…
September 4, 2020
What is Vibration Analysis?
Vibration analysis can be used to discover problems in machines and predict when they might fail. It can significantly…
Sensemore Predictive Maintenance Solution
If you enjoyed this blog, explore our Predictive Maintenance Solution page.